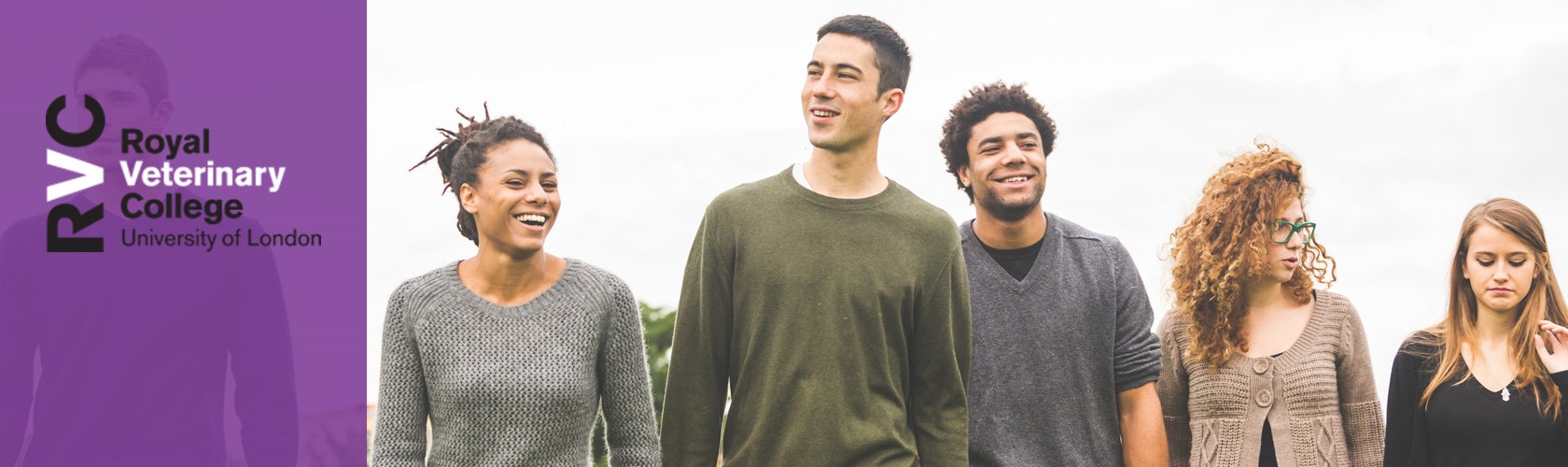
Machine learning algorithms for predicting drug resistance against tuberculosis in people
We are developing algorithms to identify novel markers and to build predictive analytical algorithms for rapid antimicrobial resistance profiling.
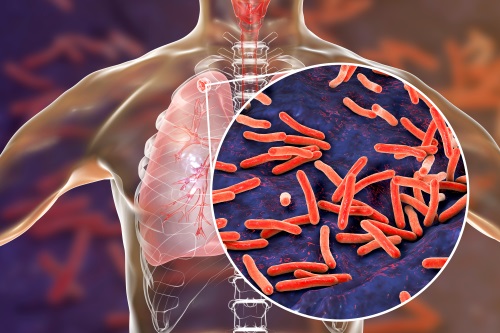
Challenge
Tuberculosis disease (TB), caused by Mycobacterium tuberculosis, is an important global public health issue, and its drug resistance, caused by genetic mutations in the M. tuberculosis genome, poses serious challenges for effective control. Current molecular diagnostic tests are imperfect as they do not target all resistance mechanisms and drugs, nor do they inform on transmission clusters, and are therefore unable to guide completely effective individualised therapy.
The full repertoire of genetic loci and mutations underpinning drug resistance is unknown. Co-occurring resistance mutations and lineage-specific mutations can be falsely identified as causative features. The application of statistical and machine learning methods to TB “big data” will need to adjust for the strong phylogeographical relationships among isolates and the structured missingness in resistance phenotypes of second line drugs.
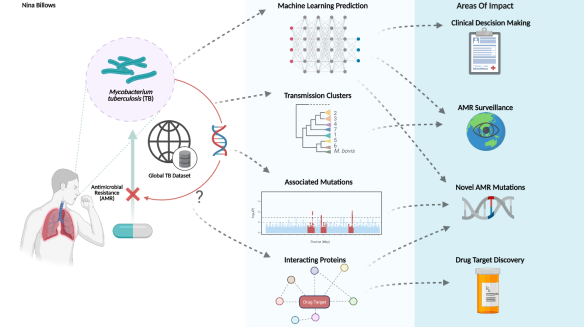
Solution
We will utilize the LSHTM 20k M. tuberculosis dataset with high quality whole genome sequencing (WGS) data and laboratory susceptibility test phenotypes across 14 drugs (generated according to World Health Organisation guidelines); these data have been sourced and curated either from a LSHTM-led global TB drug resistance study (Clark PI; >20 countries) or the public domain (years 2000 - 2019).
We will develop multi-label classification algorithms, so that resistance prediction can be done simultaneously across multiple drugs, and thereby will provide added knowledge on a complete AMR profile. We aim to apply analytical “clustering” and structured missing data imputation strategies that improve the prediction of second line drug resistance.
We will also develop a novel interaction map (a network) to characterise the complex associations between the genotypes and the phenotypes. Network mining will be applied for the hope of identifying the driving mechanism causing the resistance. These analyses will incorporate known and novel genetic pathways and functional annotations.
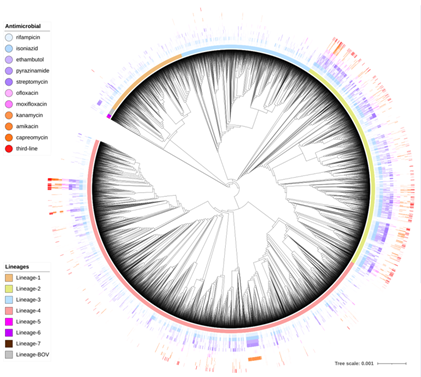
Impact
This project will have a potential impact on patient treatment decision-making. We will update the TB Profiler http://tbdr.lshtm.ac.uk/, a tool that processes raw sequence data to infer strain type and identify known drug resistance markers. We will outreach to potential stakeholders (e.g. PHE, APHA) and scope out a health economic evaluation of WGS as a tuberculosis and antimicrobial resistance diagnostic.
The strategies developed and algorithms adopted to combat the challenges in this particular study will also inform other AMR research.
The work has been funded by the RVC.
Collaborators include Professor Taane Clark and Dr Jody Phelan at LSHTM and Professor Yonghong Peng at the Manchester Metropolitan University.